Our own speculative futures at Torchbox
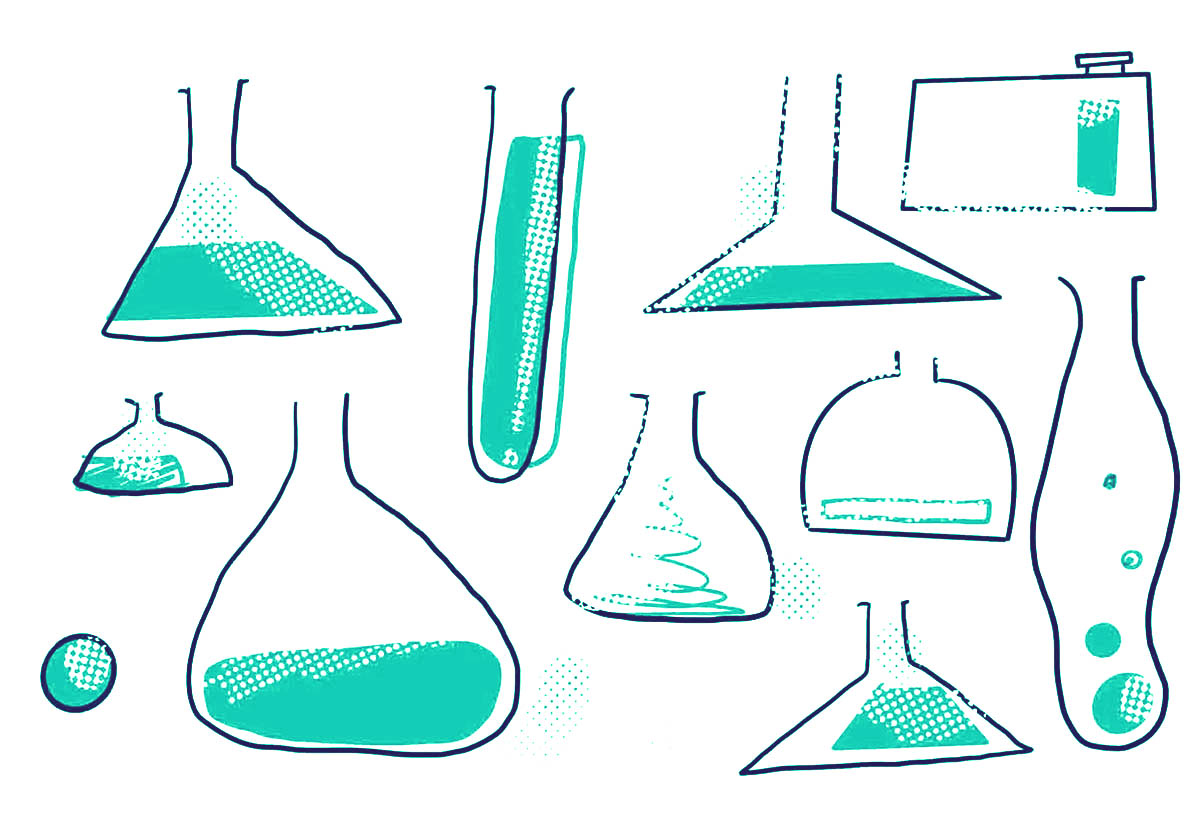
Innovation team
With the announcement of GPT-4 last week and Bard this week it’s now a question of ‘when’ things become disrupted not ‘if’. As an innovation team we created a workshop that would give us a view of possible future scenarios.
If you’re interested in working on running your own workshop you can check out our ‘Speculative Futures’ workshop guide that we’ll be posting later this week.
Sharing knowledge and how we understand these new technologies, and the possible disruptions they may create, is important. With that in mind we’re sharing back the possible futures that appear viable to use here at Torchbox alongside their probability and time-horizon.
We plotted our scenarios on a Dystopia to Utopia scale because we’ve watched too many science fiction movies. We then cheated and placed two of them in a ‘business-as-usual’ bucket. We’ll use the excuse that we’re trying to move quickly to excuse ourselves!
AI Destroys the Web
Position on scale: Pure dystopia Probability: Unlikely This would obviously be bad for a digital agency. But we’d be much more upset about losing the global commons that is the world wide web. This future could arrive if Large Language Models keep increasing the noise of already noisy spaces.
In the mid-2010s Google had a huge problem with websites keyword stuffing pages to gain better organic search positions. It wasn’t straightforward to fix but it was possible - and as a person using the internet it was also quite easy to see when a website was clearly of little value - with generative AI models that will be far harder. It could lead to people engaging with the wider web far less, moving into private digital spaces or engaging offline more. That would fundamentally alter how the web is organised and will mean organisations will need to return to pre-digital behaviours to engage their audiences.
Through the last decade we’ve had authors like Eli Pariser (The Filter Bubble), Sherry Turkle (Reclaiming Conversation) and Jonathan Haidt (The Righteous Mind) making the case against the push internet created by social media and other large digital platforms. Being reductive they argue that these platforms have reduced critical thinking and created less diverse viewpoints. Content generated by Large Language Models clearly risks exacerbating that problem not least since many have been trained on this narrow content created within social media.
It’s an unlikely future - we hope - but there is one where the web becomes so noisy that humans simply log-off.
The Darker side of AI
Position on scale: Dystopia Probability: Likely This is already happening. And it’s bad for everyone. Large Language Models reproduce human biases found across the internet. That means racism, sexism, ableism, classism and other forms of discrimination are present. And it means there are large omissions from those folk who are more likely to be excluded from the digital realm. There have been voices working to improve the situation such as Timnit Gebru, Joy Buolamwini and Annette Zimmermann. Progress has been made but it’s still possible to jailbreak the systems to write malicious content.
Even if that is solved there’s still the ‘small’ problem of how many of these Large Language Models have been populated. OpenAI stopped publishing where their data had been harvested with GPT3 but before then were heavily reliant on Cross Crawler, a 501(c) nonprofit organisation in the States. Their interpretation of Fair Use within US Copyright law has given them the ability to ingest anything available on the web. That’s problematic for many. Jaron Lanier, a computer scientist who pioneered VR in the 80s, talks about the need for ‘Data Dignity’ where AI should share sources when responding. He argues that it would acknowledge the value of individual contributions, compensate authors and make biases more visible.
With CoCo - our app to have conversations with your content - we’ve built in Jaron Lanier’s thinking from the start to acknowledge the sources of information that are being pulled in when the response is shared. It increases confidence in the data and acknowledges that the “intelligence” comes from the humans who originally contributed the content.
AI-Driven Design & Creation Dominates
Position on scale: Pretty close to utopia Probability: Likely In the late 90s there was a strange belief that Photoshop was magic. For anyone who wasn’t involved in design there seemed to be a genuine idea that designers simply pressed a button and “photoshopped” whatever we were working on. That promised land may have arrived. And if Adobe has it their way the direction to the land will be via Firefly. They’re not alone. Midjourney, Dall-E and Stable Diffusion feel like household names. Video generation is behind static image generation but not by much.
We can write essays, marketing content, emails and updates. We can experiment and iterate textual content to be longer, shorter, more formal, more upbeat or more legal. And this isn’t just for people already in the world of design and communications.
My father-in-law, who left school at 14, has suddenly been given a tool he can use to feel confident about writing a letter to the council to advocate for his neighbourhood. He was beaming when he got something that spoke with the formality that’s required to be polite but direct.
Many of these tools are already here. There’s a swarm of them. The day I wrote this, March 23, on ProductHunt there were 58 new products. 30 of them involved Large Language Models in some way and most were aiming to solve the problem of humans finding it hard to turn intangible ideas into tangible creations. Yes, the world is talking about OpenAI a lot at the moment, but there’s a relatively large level of diversity, resilience and investment going into other competitors. This design and creation space isn’t just GPT-flavoured.
AI Empowering strategy and execution
Position on scale: Utopia Probability: Likely It’s bold to predict LLMs will become even more sophisticated and move further up the hierarchy of human abilities. But we wouldn’t bet against it. In fact, we think there’s a strong possibility that in the near future LLMs will be able to support with creating experimental backlogs, better define outcomes and support strategic decision making. All that in addition to the abilities as a design and creation tool. This is the future that we’re internally preparing for through experimentation and adoption of new tools, processes and expectations of our outputs.
There are already a range of tools that have started to move up the ladder towards strategy. In user research Ask Viable automates qual data analysis, Symanto can do a pretty solid job around social listening and Kraftful will summarise reviews. For business model ideation there’s some initial greenshoots with Dimeadozen, ValidatorAI and Rationale. And if you’re needing to pitch your idea there’s now an app to automate that. Several, in, fact.
There’s some dead-ends that people are building. It’s not possible to be user-centred if you’re using AI-powered users as an obvious bad example. But the robots are coming to facilitate strategy with their tools. And as I talk about in the next speculative future tools have a remarkable way of changing all the systems around us.
Uniting people through AI
Position on scale: Pure utopia Probability: Unlikely Innovation has slowed dramatically over the last twenty years based on the impact of R&D spending at solving real-world problems. In fact there are academics arguing that peak innovation was 1873 (Heubner, 2005). Tyler Cowen, an economist at George Mason, argued in Great Stagnation that since the 1970s there’s been a declining rate of technological progress, slower growth and a shift away from productive investments. The solution to the problem could well be AI. Erik Brynjolfsson and Andrew McAfee, both MIT professors, whilst not completely agreeing to Cowen’s theory, have written extensively about how artificial intelligence can transform the economy and create space for new big ideas.
In Human Frontiers Michael Bhaskar makes the compelling argument about how these new AI tools can have the same impact that novel instruments had in the past. It’s our version of rag-based paper, the printing press or the personal computer. Over time humans have become more and more encumbered with knowledge. This is a great problem to have, but it makes finding news ideas harder. First you need to wade through all the existing work to get to the new spaces. AI looks like it could help with that. It can process, categorise, ideate and evolve. Or as Bhaskar says, ‘AI can run countless trials, prototypes, models and design adjustments, unlocking obscure perspectives.’
It could be exactly what humans need to come closer together, work across borders and cultures to produce new and incredible work.
Monorail
Position on scale: Business as usual Probability: Likely Large Language Models using Transformer-style architectures have made such incredible progress that it feels a risky move to bet against them. But they’re still not perfect and the way that they’re built may make them impossible to ‘perfect’. LLMs work by pattern matching. Based on what’s come before a statistical model is predicting what will come next. Humans can do this too with enough clues: “The best of times, the worst of …”, “In the beginning …”, “Not all who wander are …”
The problems come from across the spectrum of human thinking.
Philosophically we don’t have an agreed definition of what ‘perfect’ looks like. For business-critical websites most Service Level Agreements will demand 99.99% uptime. It’s such a valuable resource that it can only be offline for an hour a year. A Large Language Model can’t be measured in such a black-and-white way. An AI could give the ‘wrong’ answer but that might move a conversation forward. It would still have value. Equally an AI could give a ‘correct’ answer but it is so boringly correct that it isn’t particularly valuable. The same is true when generating artworks, audio files or other artefacts. The unreality of them creates a new visual space. Counterintuitively there’s a risk that as LLMs get less and less incorrect they reduce their value.
Changing fields, in maths there’s the “law of diminishing returns”. As low hanging fruit gets grabbed off of a tree it becomes harder and harder to harvest the remaining crop. Or, more formally, as you approach the limits of a system’s capacity each improvement becomes slower and more expensive. The complexity increases exponentially. In Machine Learning there’s a specific tradeoff between precision and recall. The more you increase precision - that is the fewer false positives there are - the more chance you have of reducing recall - that is the more true positives are ignored. Finding the balance between false positives and true positives whilst also giving quick responses is a monumental task. And it’s one that fully autonomous cars still haven’t solved.
We’ve written more about the fact Transformer models may never be ready for production here. But, we also think it’s only fair to say that you probably shouldn’t bet against them either.
AI gets shut down
Position on scale: Business as usual Probability: Unlikely Humans have opened Pandora’s box at this point and it seems almost impossible to shut down. Not least because there’s an array of competitors to OpenAI and Google that can be self-hosted. As of writing Stanford’s Alpaca, Dalai and Serge are all gaining stars by the minute on Github.
Regulatory oversight could increase friction for adoption though. And if Large Language Models can’t sort out their biases, or risk of misuse, then friction might be a good thing. That oversight seems more likely within the EU. Europe has already shown that it values privacy and consumer-rights through GDPR and the continuing work to reduce Big Tech monopolies. Stepping in to stop the accumulation of power that Microsoft and Google are gaining within the AI space isn’t beyond imagination.
The Climate Crisis, and cost of energy, might be a bigger risk to Large Language Models There have been a few guesstimates of how much carbon OpenAI’s models might be creating. The consensus appears to be ‘more than a google search’ but not by much. If you want to fall down a rabbit hole: Fast Company, Chris Pointin, Forbes, and Toward Data Science. In a world that is heating up though increasing our use of energy for digital needs isn’t a good place to be. But those emissions cost money and the energy is getting more expensive. Currently chatGPT is ~$0.002 for 750 words via the API or $20 / month using OpenAI’s interface. It is very hard to see how those prices won’t increase dramatically to handle the true cost of serving up the data. AI might shut itself down purely because it’s economically unviable.