Beware, AI may never be 'production' ready
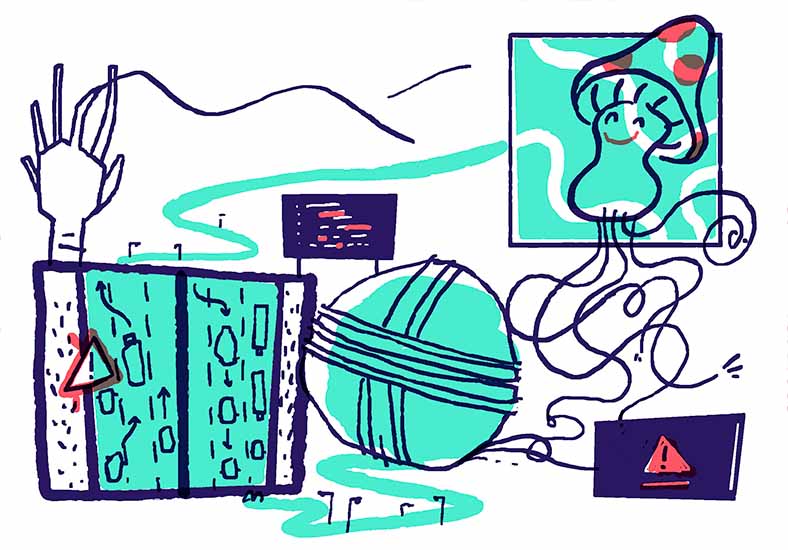
Director of Innovation
We’ve all read about how frequently OpenAI’s GPT models ‘hallucinate’. I’ve had it give me the wrong information across a range of things. From Indian Partition, through innovation frameworks to the best way to set up a Wagtail project. It even gave me a completely made up Photoshop blend mode ‘Glow’ that I really wish did exist.
It’s not lying. Lying would imply some sort of intent. But it’s also not useful and has a very large risk to do harm. It also might mean that all our concerns are for nothing. Given the progress that was made between the chatGPT3.5 model and the GPT4 model I suspect I will regret writing this in six months but:
We should be open to the idea that the hallucinations, misinformation and misrepresentation might be impossible to remove. They’re a ‘feature’ of the dataset rather than a bug.
GPT4 is a pattern creating machine. Reductively, it generates new tokens based on the probability that they should follow the tokens that have already appeared. This makes it appear like it is working magic for humans. Michael Shermer said, “Humans are pattern-seeking story-telling animals, and we are quite adept at telling stories about patterns, whether they exist or not.” We want to see a coherent and useful response from the machine and are willing to paper over the cracks when it reveals its lack of intelligence.
There was a similar amount of hype around self-driving cars in the late 2010s. Through 2016 and 2017 self-driving technology appeared to be months away from production. There was only “1%” left to sort. But it was an illusion. The cars weren’t driving “themselves”, they were following a highly detailed pattern in fairly safely controlled environments with LIDAR - and other sensors - to control for the unexpected. We’re still a long way from truly autonomous vehicles, though we do now have the joy of many YouTube videos showing cars getting stuck and then running away when the humans show up.
That final “1%” is hard to get over. Domestic robots are still idiots. Voice assistants are only useful for setting timers and briefly distracting kids. Visual sentiment analysis - where a machine would be able to tell if someone is happy or not - has proven to be a dead-end.
There’s a paradox here. Literally. Hans Moravec came up with it back in the 90s. Moravec’s Paradox is that things that are below our level of conscious awareness, or have a very low cognitive load for humans, are exceptionally hard for computers to handle. High cognitive load tasks - playing chess, doing calculus - are easier. His hypothesis was that it related to biological evolution. Or as Steven Pinker said more succinctly,“the main lesson of… AI research is that the hard problems are easy and the easy problems are hard.” Awkwardly, that’s a quote from 30 years ago as well.
It’s a risky prediction to make but one viable future is that OpenAI, Google, Microsoft et al aren’t able to make it to the point where their models are reliable 99.9999% of the time. It’s very hard to make it over that final hurdle and always takes longer than expected.
Back in the 1970s Hofstadter’s Law was coined. The law: “It always takes longer than you expect, even when you take into account Hofstadter’s Law”. It might not be a matter of days but of decades. Hofstadter coined his eponymous law because of his frustration at a computer being unable to beat a human at chess. In the 1970s that felt frustratingly close considering the depth of analysis a computer could make but was forever out of reach since computers kept getting beaten. It was another 20 years before Kasparov was taken down by IBM.
OpenAI’s models are having a bigger splash than self-driving cars - and even DeepBlue - for two reasons. First, many more of us feel threatened by this invention. If someone is able to go and ask a machine to design them an innovation workshop do I have a job anymore? Second, because the model is so accessible it’s easy for us to play with it. It feels tangible - and close - in a way that watching a Waymo car doesn’t.
On a similar wavelength to Michael Shermer, Stephen Jay Gould - a biologist and science historian - wrote, “We are story-telling creatures… But our strong desire to identify trends often leads us to detect a directionality that doesn’t exist, or to infer causes that cannot be sustained.” The story of how Large Language Models are going to disrupt whole industries is a gripping one. It’s terrifying - and a little exciting - but at the moment it’s just that, a story. It’s worth considering that we’re doing a really good job at telling one another a convincing story and that it’s pointing us in the wrong direction.